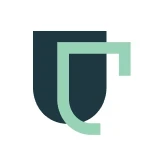
Franco Brutti
Have you ever heard of data mesh?
Data mesh has already become a crucial concept in data science at all scales. But it has also become quite a valuable resource in IT and certainly in the marketing world.
This approach is key when it comes to managing large-scale data, so it has already become a vital piece for many organizations. Therefore, if you are looking to specialize in data management, this will be an indispensable tool for you.
And for this reason, we will tell you what data mesh is, where you can apply it and what its benefits are.
What is data mesh?
The term "data mesh" has become paramount in the field of data management and data engineering in recent years.
It’s an architectural and organizational approach to manage and democratize access to data in enterprises.
Data mesh is based on data decentralization. In other words, instead of having a central data management team, the data mesh approach involves decentralizing data ownership.
As a result, data is managed in a distributed manner throughout the organization, and each team or domain is responsible for its own data.
Moreover, data is grouped into what are called data domains. Each domain is a logical unit that is responsible for its own data, its quality and its availability.
However, each data domain or platform is considered a product within the data mesh.
In other words, instead of treating data management as a centralized service, it’s considered a product. Each data domain is responsible for providing a data platform that enables other teams to access and use their data effectively.
And to facilitate access to data and the integration of the different platforms without relying on a central system, APIs are essential.
The importance of data mesh
Data mesh addresses some of the key challenges organizations face in the era of big data and digitization for the following reasons:
Scalability: as organizations accumulate large volumes of data, centralized data management infrastructure can become unsustainable. Data mesh allows data management to scale in a distributed manner, making it easier to manage large amounts of information.
Agility: The data mesh approach allows teams and data domains to be more agile in managing and accessing data. This means that organizations can adapt more quickly to changing business needs.
Data democratization: by decentralizing data ownership and providing autonomous access through APIs, data mesh promotes data democratization. This means that more people within the organization can access and use data efficiently.
Uses and applications of data mesh
Enterprises and corporations: Organizations, agencies and corporations as well as medium-sized companies with multiple teams can use data mesh to manage their data. And of course, to create and establish scalable management models.
Technology and startups: to make decisions and improve products and services, they can benefit from the implementation of a data mesh from the beginning. This allows them to scale their data infrastructure as they grow.
Healthcare industry: in the healthcare sector, data management is essential for patient care, medical research and service improvement. Data mesh can help manage patient data, electronic medical records and other critical data more efficiently.
Media and entertainment: Media and entertainment companies generate countless amounts of data on user behavior, preferences and content. Data mesh can help manage and analyze this data to deliver much more personalized and accurate experiences to users.
E-commerce: A data mesh can help improve inventory management, personalization and merchandising-related decision making.
Transportation and logistics: In the transportation and logistics industry, data management is critical for shipment tracking, fleet management and route optimization. Data mesh can help coordinate and effectively leverage real-time data.
Government and public sector: A data mesh approach can improve efficiency and transparency in the delivery of public services. Governments and public sector organizations handle a large amount of data related to public administration, health, education and other services.
Data mesh techniques
You have a wide range of techniques and practices when implementing data mesh. However, these are some of the most preferred among specialists:
Data domains: that is, defining and creating clear and specific data domains within the organization. A data domain is a logical set of related data that are managed and are the responsibility of a specific team or area in the company.
Data platform as a product: this technique is based on viewing the data infrastructure as a product that is offered to internal users; not as a centralized service. This involves building and maintaining a data platform that is easy to use and provides teams with the tools they need to manage and share their data.
APIs and autonomous data access: establishing APIs to allow teams to access data from other domains autonomously. This involves creating standard and secure interfaces for data communication between teams.
Data quality and governance: this practice consists of establishing data quality policies and standards, as well as governance practices to ensure data integrity, security and privacy. Each data domain is responsible for complying with these policies.
Data catalog: a centralized data catalog that allows users to search and discover data sets available in the organization. This makes it easier to locate and access data.
Observability and monitoring: to monitor the performance of the data infrastructure and ensure that data is available and reliable at all times.
Automation and DevOps: this technique consists of using automation practices and DevOps principles to streamline the development and deployment of data infrastructure and software updates.
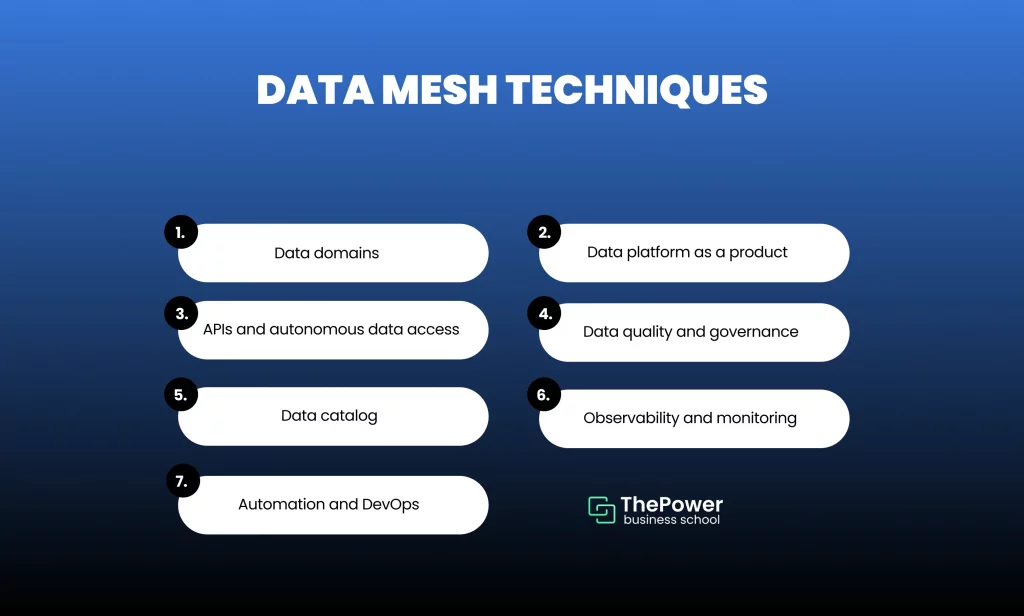
Benefits of data mesh
These are some of the major advantages of data mesh for your organization, whether you are an SME, startup or large enterprise:
Agility: teams and data domains can be more agile in managing and accessing data. This makes it easier to adapt to changing business needs and respond quickly to opportunities and challenges.
Data democratization: data mesh promotes the democratization of data by making it available and accessible to a broader set of people within the organization. This encourages informed decision making at all levels.
Improved data quality: each data domain is responsible for the quality of its data, which can lead to an overall improvement in data quality across the organization. This is essential for accurate and reliable decision making.
Bottleneck reduction: By avoiding congestion in a central data management team, data mesh can reduce bottlenecks and delays in data access, which improves operational efficiency.
Scalability: data mesh allows data management to scale more effectively as the organization grows and accumulates more data. By decentralizing responsibility for data, bottlenecks common to centralized approaches are avoided.
Technology flexibility: data mesh is not tied to a specific technology or tool, which means that organizations can use the technologies that best suit their needs and environments.
Disadvantages to consider
Let us now look at some of the possible disadvantages of data mesh in practice:
Initial complexity: implementing data mesh can be complex and time-consuming, especially if the organization already has an established data infrastructure. It requires significant planning and investment in terms of human and technological resources.
Coordination and governance: Coordinating multiple data domains and ensuring data consistency, quality and security can be a challenge. Lack of adequate governance can lead to inconsistent data issues and duplication of effort.
Cultural change: transitioning to a data mesh approach may require a cultural change in the organization, as it involves greater accountability and collaboration across teams. This can be difficult to achieve in organizations with a traditional data-centric culture.
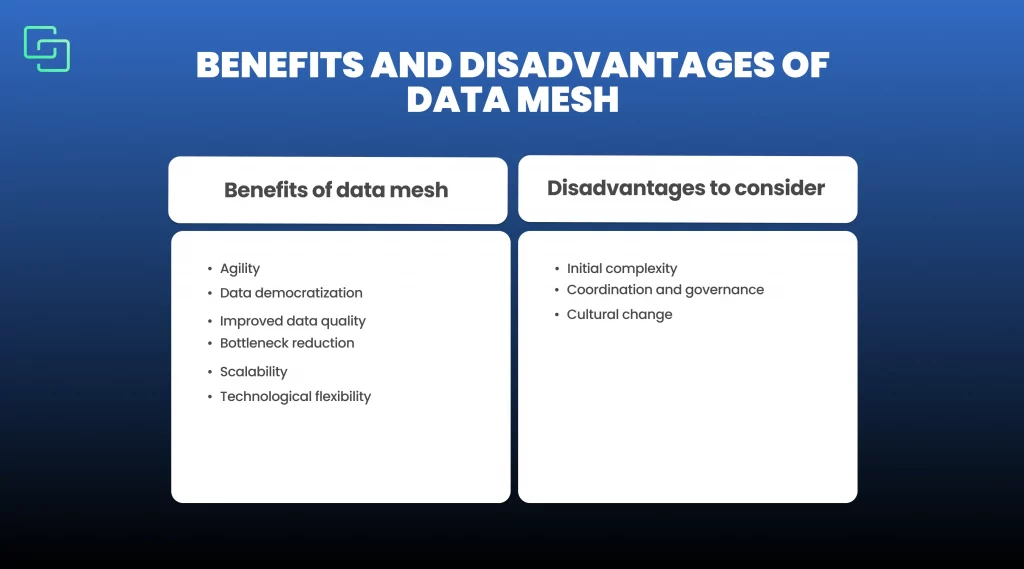
Data mesh, data lake & data warehouse: differences and key points
Data mesh is very, very closely related to data warehouses and data lakes, but represents a different approach to managing and organizing data within an organization. Here's how they are related to each other and how they differ from one another:
1. Data Warehouse
A data warehouse is a centralized, highly structured database used to store historical and processed data for business analytics.
Data warehouses are typically designed to consolidate data from multiple sources to create a single, much more complete, global information source. Data mesh focuses on data decentralization and data accountability.
In a data mesh approach, instead of consolidating all data into a single warehouse, data is distributed and managed in separate data domains, each with its own infrastructure and accountability.
Data warehouses can be part of a data mesh. But remember: data mesh promotes collaboration across data domains and democratization of access.
2. Data Lake
A data lake is a data repository that stores data in its raw, unprocessed and unstructured format.
Data lakes are known for their ability to handle large volumes of data of different types and formats. In data mesh, data lakes can still be used to store data, but the key difference is how that data is managed and accessed.
Instead of being a centralized warehouse, the data in a data mesh is divided into data domains with their own access policies and APIs, allowing teams to access and manage their data autonomously.
Data mesh, when combined with other approaches to data science and data management, can become a fundamental building block for your strategies and projects, no matter their scale.
This approach will help you segment each and every one of the different data structures and accesses within your company. It will also help you improve communication and, above all, collaboration between teams.
Thus, you will take your data systems and management strategies to the next level. And this will translate into better results for your projects.
And now, we want to know the most important opinion: yours. So tell us your perspectives and opinions in the comments.
Oct 14, 2022